Speaker: Prof. Benoît Macq.
November 21, 2019 (French)
Venue: Radiology Department – Cliniques Universitaire St. Luc
The images make it possible to diagnose a situation, to develop an action plan and to drive and validate the execution of this plan. In medical physics, image acquisition models allow partial prediction of the biological features. In signal processing, algorithms transform the images into visual features, such as contours, textures and topological properties, that are understandable by humans, while in machine learning the features are learned from large data sets annotated by experts to get the best predictive performances. In the above three approaches, the image to analyse is projected onto a latent space (the features space) of reduced dimensionality suitable for taking efficient decisions. As the search for more powerful algorithms progresses, the difficulty of interpreting results from complex predictive models based on high-order interactions between many variables creates difficulties of mutual understanding between domain experts and experts in signal processing and artificial intelligence.
The goal of this research proposal is to address this issue by grouping entities, including data providers and domain experts, in integrative coalitions sharing common algorithms with the following quantitative and qualitative gains:
- increased reliability and explainability of decisions
- privacy and measurable quality of data
- measurable trusted co-evolution of algorithms and domain experts.
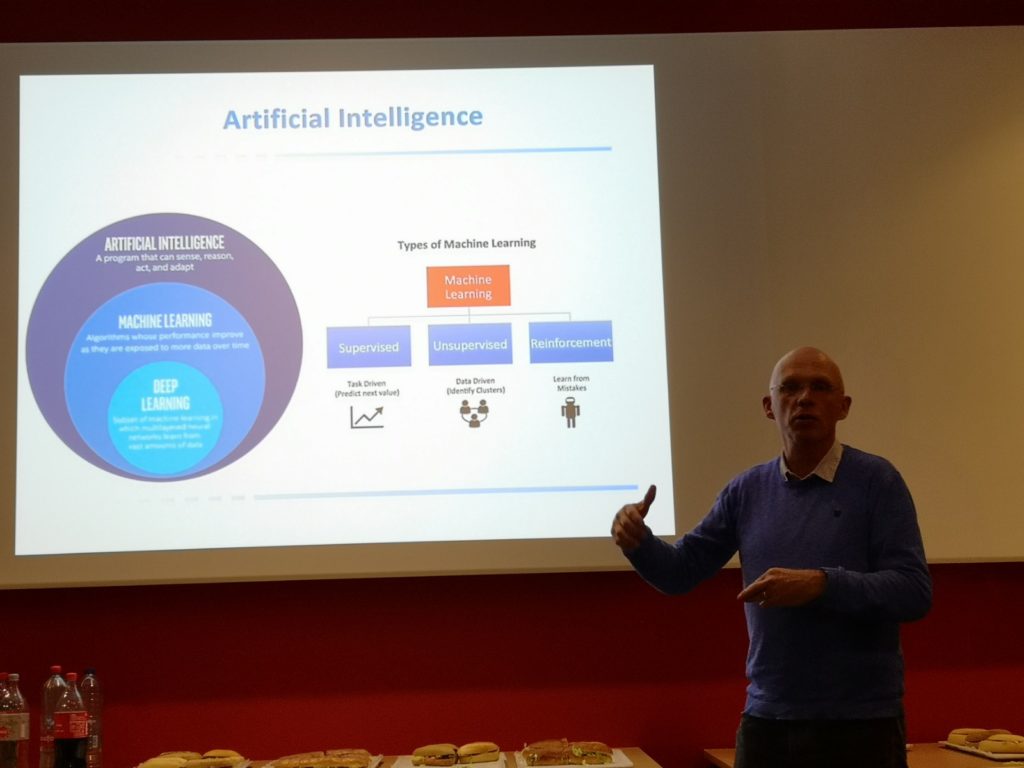
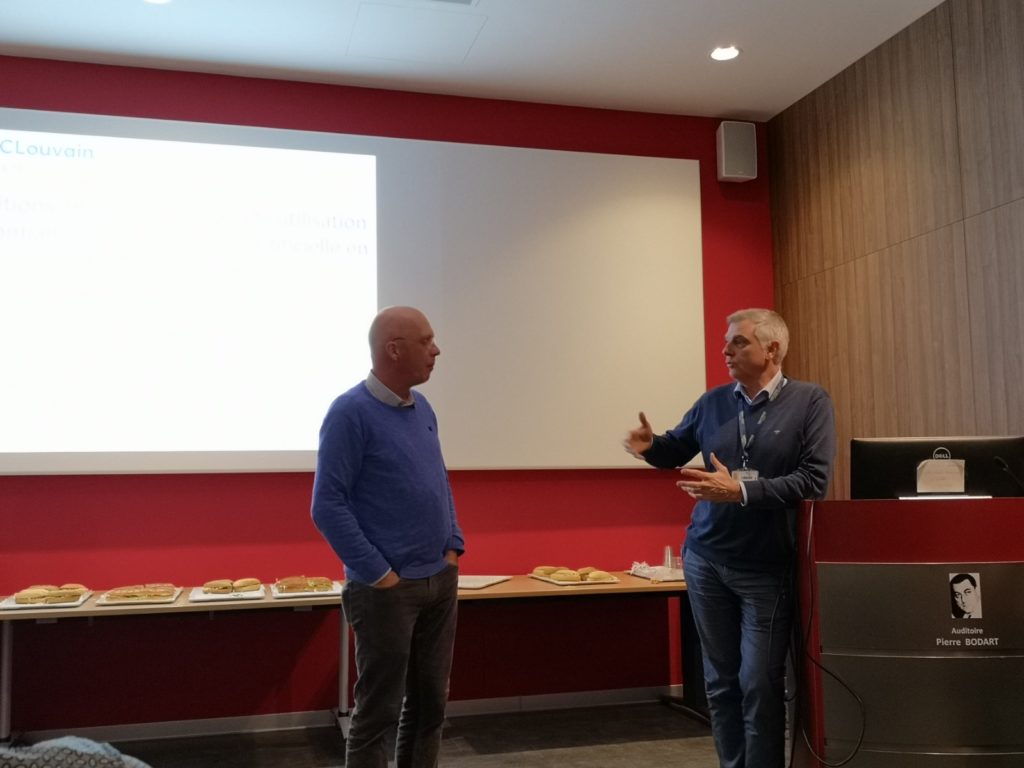
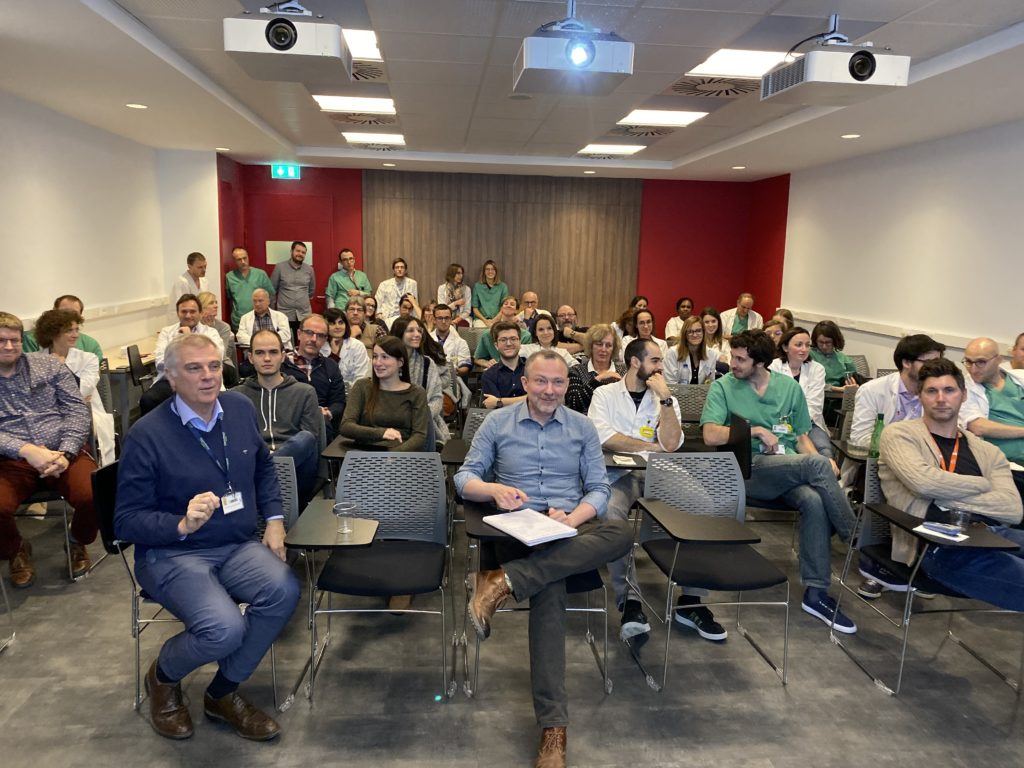