Every month, LIAM highlights a researcher to keep people up-to-date with new research development as well as reflections on AI for medicine.
Researcher of the month
May 2021 – Gaëtan Rensonnet
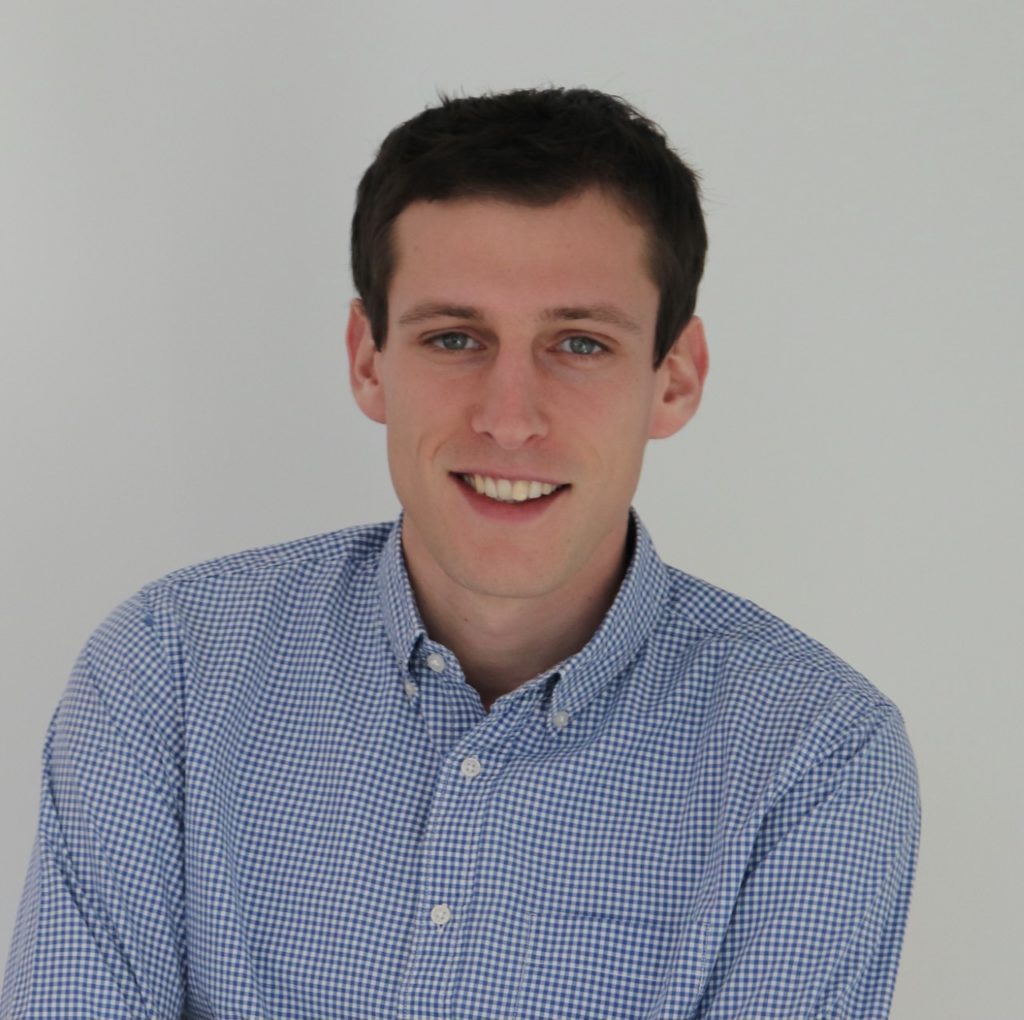
Gaëtan Rensonnet obtained his PhD in electrical engineering jointly from Université catholique de Louvain (UCLouvain) and Ecole polytechnique fédérale de Lausanne (EPFL).
His research aims to increase the clinical value of non-invasive brain imaging by leveraging signal processing, numerical simulations and artificial intelligence. He is currently a post-doctoral researcher at ICTEAM in Prof. Benoît Macq’s team. He is involved in several population studies of brain disorders and is also acting as project manager for the recently created Trusted AI Labs (TRAIL) institute, which promotes AI research and its transfer to the local industry in a number of fields including health care.
The common denominator of the various ongoing population studies at PiLab is the link between clinical outcomes and structural changes in the brain white matter (WM) at the microscopic level, which is referred to as WM microstructure. Two examples of research projects are described below.
Motor recovery
Collaboration with Prof. Y. Bleyenheuft, Prof. Y. Vandermeeren, Dr Ir L. Dricot (IoNS).
As part of the MicroMoto Action de Recherche Concertée (ARC), a novel intensive neurorehabilitation approach, known as HABIT-ILE, is being tested in two very different populations: infants born with bilateral cerebral palsy and adults suffering from chronic motor impairment after a stroke (Figure 1). Using the recently installed high-gradient MRI scanner at Cliniques Universitaires Saint Luc, we acquired advanced multi-shell diffusion-weighted MRI for all subjects. Our microstructural markers obtained from these images will allow us to shed light on the WM tracts and regions involved in motor recovery and to predict the chances of recovery (Figure 1).
Automatic psychiatric diagnoses
Collaboration with Dr Ir M. Taquet (Oxford University), Prof. S.K. Warfield (Harvard Medical School).
This project tackles the problem of label uncertainty in very large-scale population studies of psychiatric disorders such as the UK Biobank and the Adolescent Brain Cognitive Development study (ABCD). Psychiatric diagnoses are notoriously complex as they depend on multiple factors and cannot always rely on established biomarkers (imaging, blood analysis, etc.). Additionally, it is not practical to have each subject examined by a trained clinician in large-scale population studies. Diagnoses or “labels” in such studies are thus only available indirectly, for instance via patients’ self-reporting or questionnaires. In this project, our indices of the brain WM will be combined with multi-modal markers and a machine-learning model will be trained to predict psychiatric diagnoses such as depression or bipolarity (Figure 2). The model will also learn the uncertainty on each label (confusion matrices) and output confidence levels for each psychiatric disorder and each diagnosis method.
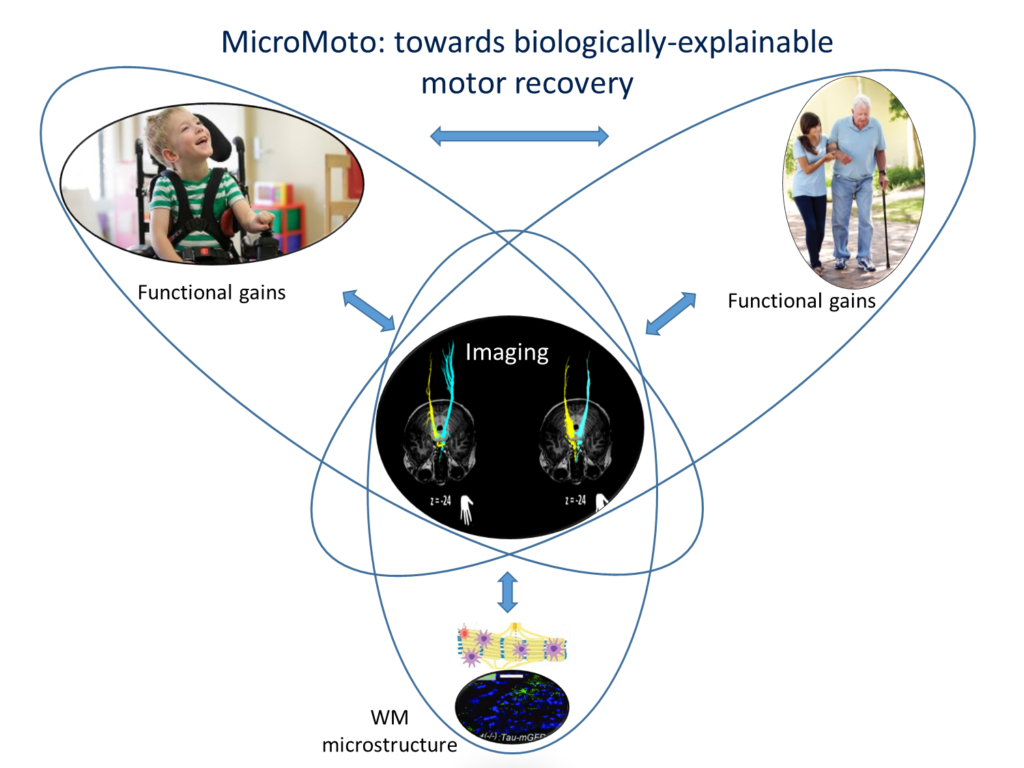
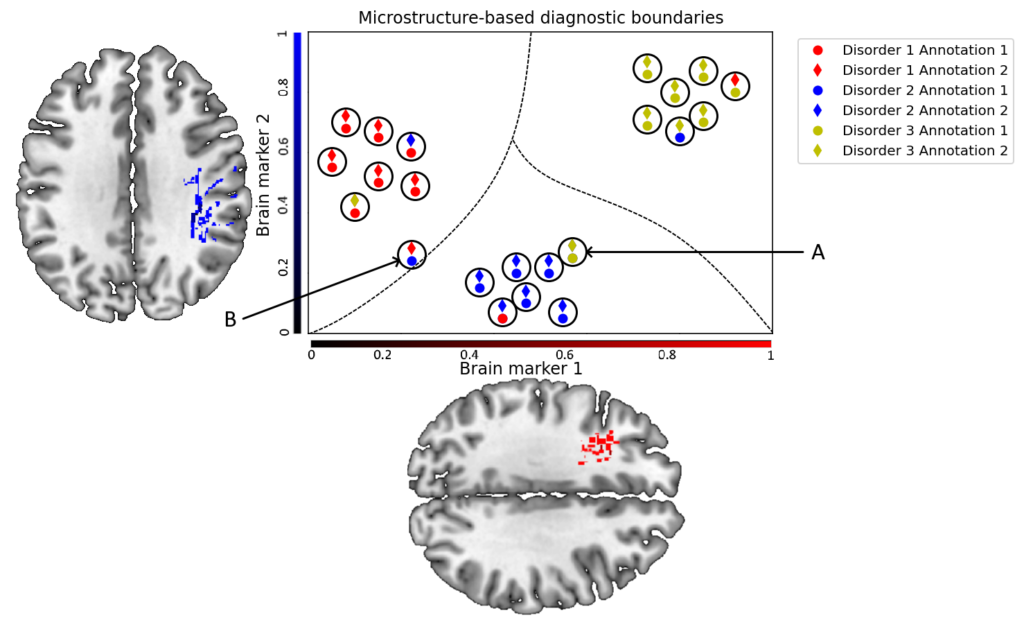