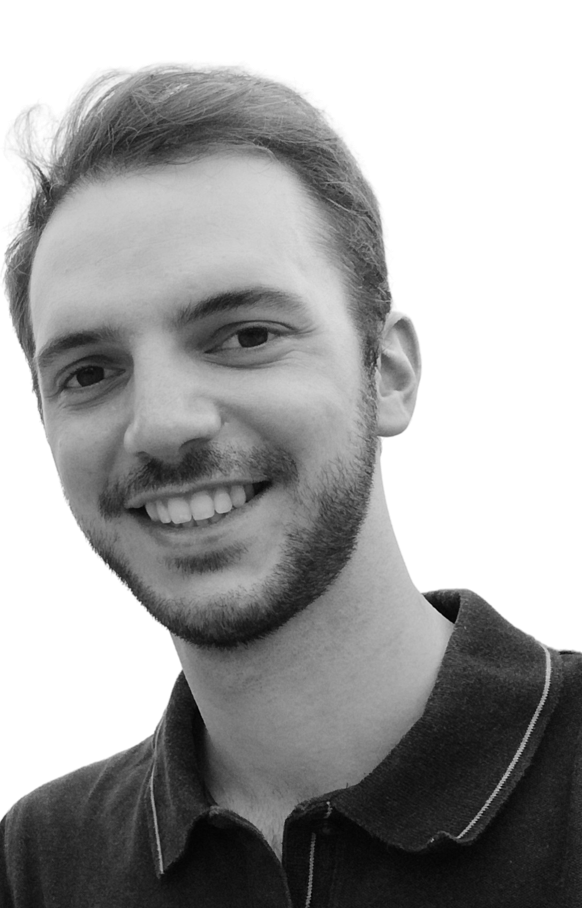
Jean Léger received his Electrical Engineering Master’s degree from UCLouvain in 2016. He is currently F.R.S.-FNRS Research Fellow completing a PhD under the supervision of Prof. B. Macq and Prof. C. De Vleeschouwer. His research focuses on image segmentation with deep learning for biomedical applications. In such applications, datasets are often “imperfect” since annotations (and sometimes also data) required for supervised learning are hard to collect. Hence, the annotations are often scarce and/or noisy.
DEEP LEARNING-BASED SEGMENTATION OF MINERALIZED CARTILAGE AND BONE IN HIGH-RESOLUTION MICRO-CT IMAGES
One of Jean’s research projects aims at automatically segmenting mineralized cartilage from bone in micro-CT images (collaboration with Prof. G. Kerckhofs). Because of its function of mediating load between very dissimilar tissues, the bone-to-tendon interface is a common site of injury. However, in case of such injury, the natural tissue is not regenerated after healing. Instead, it is replaced with a so-called scar tissue, which is isotropic and significantly less stiff than either tendon or bone. It has been reported that, depending on the severity and the location of the injury, the regenerated tissue will rupture again in 20 to 94% of cases. A solution to this problem would be to use an artificial construct to obtain a tissue that presents the same properties as the original one. In this context, characterizing the bone-to-tendon interface properties and its 3D sub-architecture is essential. High-resolution 3D micro-CT imaging is a powerful tool for the visualization of the mineralized tissues. However, it remains challenging to discriminate automatically between mineralized cartilage and bone as they have similar greyscale values. Currently, manual contouring is still the standard way to segment these two tissues but it is time-consuming and user-biased.
In this project, a 3D fully convolutional neural network (i.e. U-net) was optimized to automatically segment mineralized cartilage from bone in high-resolution micro-CT images of the Achilles tendon-to-bone interface. Using 3D U-net, an average Dice Similarity Coefficient (DSC) of 0.85 was reached compared to manual annotations for twelve 3D datasets (see Figure). The proposed method shows comparable results to a 2D U-net approach in terms on DSC while ensuring better 3D segmentation consistency. Current research aims at measuring the model confidence in its prediction in order to better interpret and/or improve the automatic segmentation. Semi-supervised and unsupervised approaches are also studied to lower the amount of annotated images required for training.
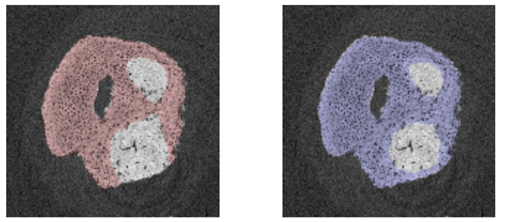