Pietro Maggi is a neurologist at the Cliniques universtaires Saint-Luc affiliated to the Institute Of NeuroScience at UCLouvain. He is particularly interested in improving the diagnosis and prognosis of brain and spinal cord inflammatory diseases using advanced imaging techniques.
IMPROVING THE DIFFERENTIAL DIAGNOSIS OF MULTIPLE SCLEROSIS USING ADVANCED IMAGING
Multiple Sclerosis (MS) is a chronic immune mediated disease of the central nervous system (CNS) characterized by focal inflammatory lesions in the brain and spinal cord. Magnetic resonance imaging (MRI) offers the unique opportunity to observe in vivo the formation and development of CNS inflammatory lesions and is an invaluable tool for MS diagnosis. Although highly useful, the MRI techniques commonly used in the clinic have a specificity that is too low to reveal the underlying brain pathology which results in i) misdiagnosing MS in individuals affected by other disorders, ii) underestimating MS immunopathological heterogeneity and iii) limited accuracy in the prediction of conversion to MS in “at risk” individuals. Overall, this hampers the use of MRI to guide individually tailored treatment.
With his team, Dr Maggi has recently shown that novel MRI techniques, featuring higher specificity for MS pathology, can significantly improve the differential diagnosis between MS and other diseases mimicking MS (“MS mimics”; Maggi et al. Ann Neurol 2018; Maggi et al Ann Neurol 2020), and that these novel MRI features can be efficiently detected using a faster non-operator dependent deep-learning approach (Maggi et al NMR Biomed 2020; Barquero et al. NICL 2020). Their pioneer methods are based on 3D patch-based convolutional neural network (CNN) architecture, exploiting several MRI contrasts combined at the first and last layers of the network. Although giving promising results, these methods require manual image processing steps (e.g. manual brain lesion pre-selection), and fully automated and longitudinally validated pipelines are currently under investigation (Huelnhagen et al. ISMRM 2021, submitted; Wynen et al. ISMRM 2021, submitted).
Thanks to the collaboration between the Departments of Neurology, Radiology (at the Cliniques universitaires Saint-Luc) and the LIAM, they now aim at integrating advanced and established MRI features with clinical and biological information to implement a novel multimodal diagnostic framework for MS (see Figure). A machine learning approach using clinical, laboratory and MRI information will be trained and used to predict MS vs. MS mimic clinical diagnosis (using supervised classifier) and to identify differences within the MS cohort with regards to clinical, laboratory and radiological features (using non-supervised classifier with clustering approach).
Once validated, this novel multimodal diagnostic framework will be used to predict conversion to MS in patients at risk for developing the disease. Overall, this project has the potential to reduce diagnostic errors, providing new tools for personalized diagnostic and therapeutic decision-making of CNS immune mediated diseases.
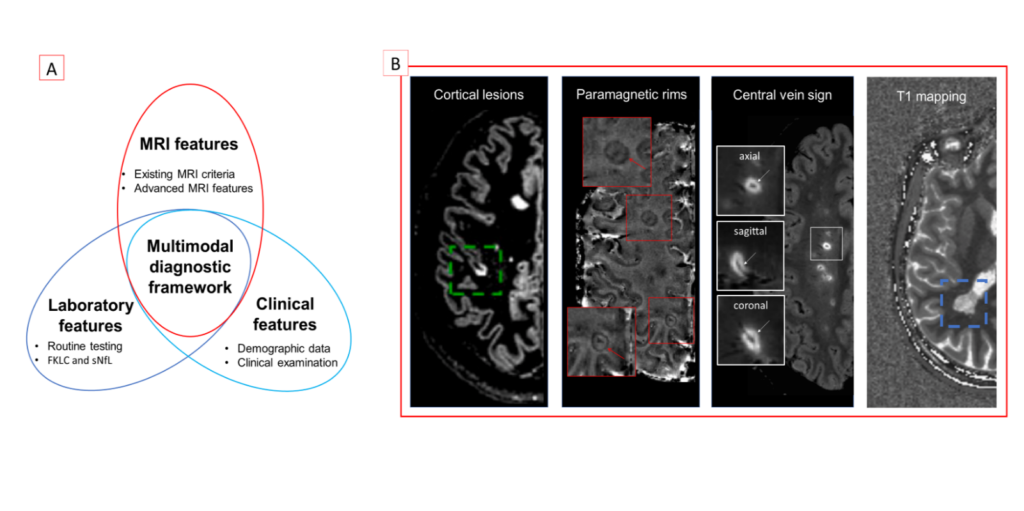