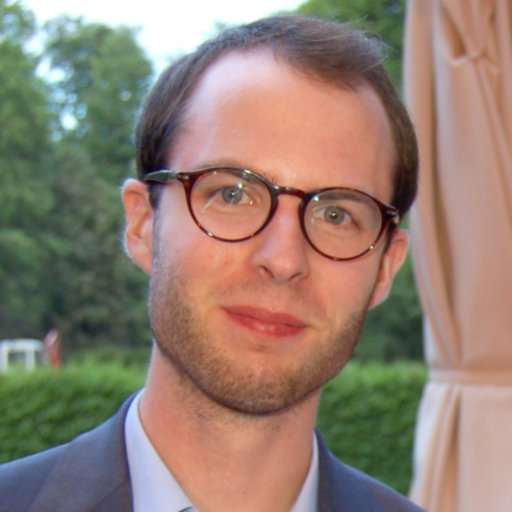
Eliott Brion has a master degree in Applied Mathematics jointly from UCLouvain and CentraleSupélec. He is currently pursuing the Ph.D. in Applied Science with UCLouvain, with a focus on deep learning for radiotherapy, supervised by Prof. B. Macq and Prof. J. Lee.
MEASURING ANATOMICAL VARIATIONS BETWEEN RADIOTHERAPY TREATMENT SESSIONS TO IMPROVE DOSE CONFORMITY
Radiotherapy consists in delivering a prescribed dose, typically X-rays, to the tumor, while limiting the dose delivered to the surrounding healthy organs. Delivering precise dose to the tumor is key to destroy it and avoid giving dose to healthy organs is important to prevent secondary effects (e.g., bleeding rectum) or prevent causing a new cancer in these areas.
During the treatment, the dose is not delivered at once but is divided in several small doses called fractions (e.g., one fraction a day for 20 days). For prostate cancer patients, large organ deformations occurring between radiotherapy treatment sessions create uncertainty about the dose delivered to the tumor and surrounding healthy organs. Automatically identifying those structures on treatment day would help improving dose conformity across treatment sessions. In his latest work, Eliott and the team trained a 3D U-net deep-learning network to segment bladder, rectum and prostate on on cone beam CT (CBCT) scans that are acquired on each treatment day (and only used for patient positioning at this time). Due to the scarcity of contoured CBCT scans, they augmented the training set with CT scans already contoured in the current clinical workflow and tested the newly trained network on CBCT scans. The segmentation accuracy (as measured by the Dice similarity coefficient) increased significantly with the number of CBCT and CT scans in the training set, reaching a performance 10% better than conventional approaches based on deformable image registration between planning CT and treatment CBCT scans. Interestingly, adding CT scans to the CBCT training set allowed maintaining high performance, while halving the number of CBCT scans. Hence, this work showed that although CBCT scans included artifacts, cross-domain augmentation of the training set was effective and could rely on large datasets available for planning CT scans.
This work demonstrates that the proposed approach (enlarging the training set with CTs) allows to be more precise when automatically contouring CBCTs. In the future, this could be used to measure and ultimately reduce the dose delivered to healthy organs. A next step will be to highlight the impact of such technique to allow better tumor coverage and reduction in the doses delivered to the healthy organs.
To know more about this project, you can read the corresponding publication here.
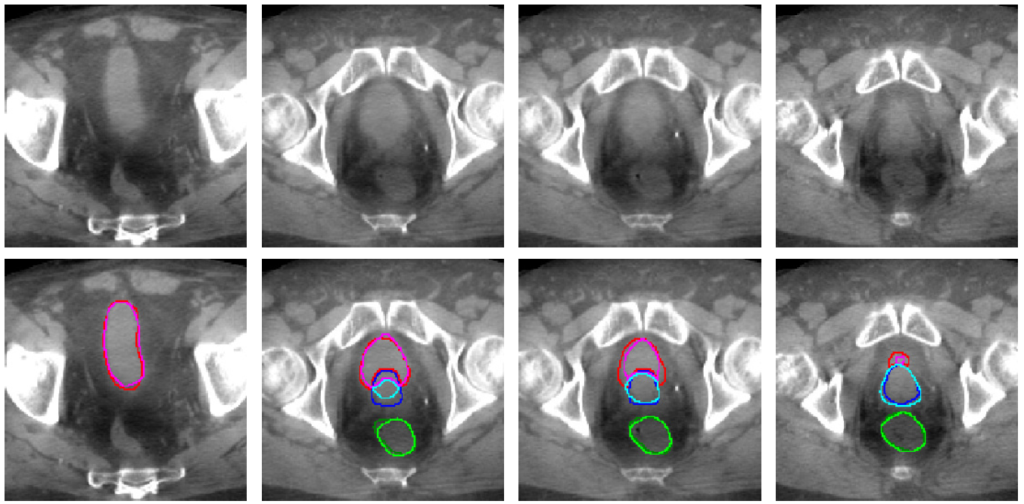