The laboratory of Molecular Imaging, Radiotherapy, and Oncology (MIRO) works on a variety of projects that combine technical and clinical knowledge with the aim of improving radiation oncology treatments. Radiation therapy is one of the most used modalities to treat cancer and it uses different types of medical images, like computed tomography (CT), positron emission tomography (PET), or magnetic resonance images (MRI), for diagnosis, treatment planning, treatment delivery, treatment verification, and follow-up. Joining the recent rise of artificial intelligence methods applied to the medical domain, the MIRO laboratory is exploring different ways of applying deep learning tools to improve and automate radiation therapy treatments.
Meet the members of the MIRO team and read about their success stories!
Umair Javaid and Kevin Souris work together to mitigate inherent noise in Monte Carlo dose distributions using deep learning[1]. In particular, they use a U‐Net architecture with dilated convolution kernels (U7 in Figure 1) to denoise dose distributions for proton therapy treatments calculated with a Monte Carlo dose engine [2]. Denoising of dose maps has two advantages: first, it smooths the dose maps, which eases visual inspection by physicians in clinical routine, and, second, it reduces the number of particles to be simulated, thus, accelerating the dose calculation process.
In addition, Umair also works on deep learning models for automatic segmentation of organs from CT images, applying also a U-Net architecture [3, 4].
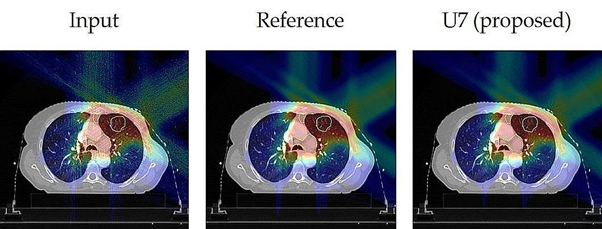
Figure 1. Corresponding slices of the dose distributions taken from a lung test case, showing the noisy Input, the nearly noise-free Reference, and the result after denoising with the proposed U-Net model (U7).
Steven Michiels, Sara Teruel and Ana Barragán join forces to tackle the challenge of treatment plan automation and standardization. Treatment planning in radiation therapy is a long and complex task, and thus, in order to ensure fast and high quality treatment planning for all patients, the process must be automated and standardized. As part of the CHARP project funded by the Walloon region and in collaboration with the ICTEAM group from Louvain-la-Neuve, Steven, Sara, and Ana try to apply deep neural networks to directly predict a patient-specific optimal dose distribution [5] (Figure 2).
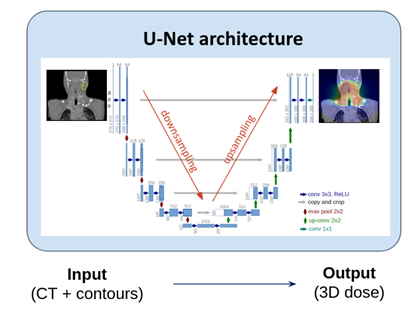
Figure 2. Deep neural network to predict a 3D dose distribution from a patient’s anatomy (CT and contours for target and organs volumes), using a U-Net architecture.
Prof. John A. Lee, head of the MIRO lab, is supervisor of all these projects and some others that address more fundamental questions of machine learning (dimensionality reduction) and image processing (deconvolution).
Prof. Edmond Sterpin, is also co-supervisor of the above mentioned projects and works with the European SocieTy of Radiation Oncology (ESTRO) to organise lectures to teach clinicians about deep learning tools.