Because it is sensitive to the microscopic displacements of water molecules diffusing in the tissues, diffusion-weighted magnetic resonance imaging (DW-MRI) has become the modality of choice to study the anatomy of the white matter at the micrometer level, with applications in the diagnosis and treatment of many neurological disorders and brain injuries. Our new approach at the intersection of physical modeling, numerical simulations and signal processing proposes a way to bridge the gap between the native millimeter resolution of MRI and neuroanatomical indices at the micrometer scale.
We use specific MRI acquisitions known as diffusion-weighted MRI which by design are sensitive to the micrometer-scale diffusing motion of water molecules in the tissues at body temperature. In traditional approaches, forward mathematical models based on analytical formulas are devised to link the geometry of white matter tissue, the diffusion of water molecules in that geometric arrangement and the physics of MRI acquisitions. The free parameters of such a forward model are then adjusted to new MRI measurements by minimizing a cost function, providing indices of the white matter microstructure locally in each voxel. Such indices usually reflect the morphometry of the axons and glial cells making up the white matter.
In our latest work, we presented a forward model in which the MRI signal is not modeled analytically but instead computed by numerical simulations of the random walk of water in a 3D mesh representing the tissue. Such simulations, known as Monte Carlo simulations, provide signals that are more physically accurate than most traditional closed-form analytical models used in the literature. Due to their long computation times, their use has been mainly restricted to validation rather than directly in a model of the signal. In addition, being numerical results rather than mathematical formulas, they do not easily lend themselves to continuous optimization frameworks for parameter estimation. To circumvent these difficulties, we made use of combinatorial and convex optimization on a presimulated collection of MRI signals, or fingerprints, wherein each fingerprint uniquely characterizes a typical microstructural configuration.
On a rat model of spinal cord injury, we showed that the microstructural indices obtained with our model based on in vivo, non-invasive MRI were consistent with histological observations performed after sacrificing the animal. In particular, our model was able to detect axonal loss in the injured part of the spinal cord. The method was then applied on in vivo human brain data. Indices of axon diameter and density in specific white matter pathways were found to agree with previous histological observations in the human brain.
Future work will focus on using 3D geometries which more closely mimic white matter tissue in our presimulated dictionary of fingerprints. The method will also be applied on existing clinical datasets. This work encourages the use of computational models of the diffusion signal and gets us a step closer to quantitative characterization of the white matter microstructure in vivo.
Collaborations
Signal Processing Lab (LTS5), Ecole polytechnique fédérale de Lausanne, Switzerland
Computational Radiology Laboratory, Boston Children’s Hospital, Harvard Medical School, Boston, USA
Institute of NeuroScience, Université catholique de Louvain (Mont-Godinne), Belgium
Links
Publisher: https://www.sciencedirect.com/science/article/pii/S1053811918319487
Twitter (@MaximeTaquet): https://twitter.com/MaximeTaquet/status/1048534818831646722
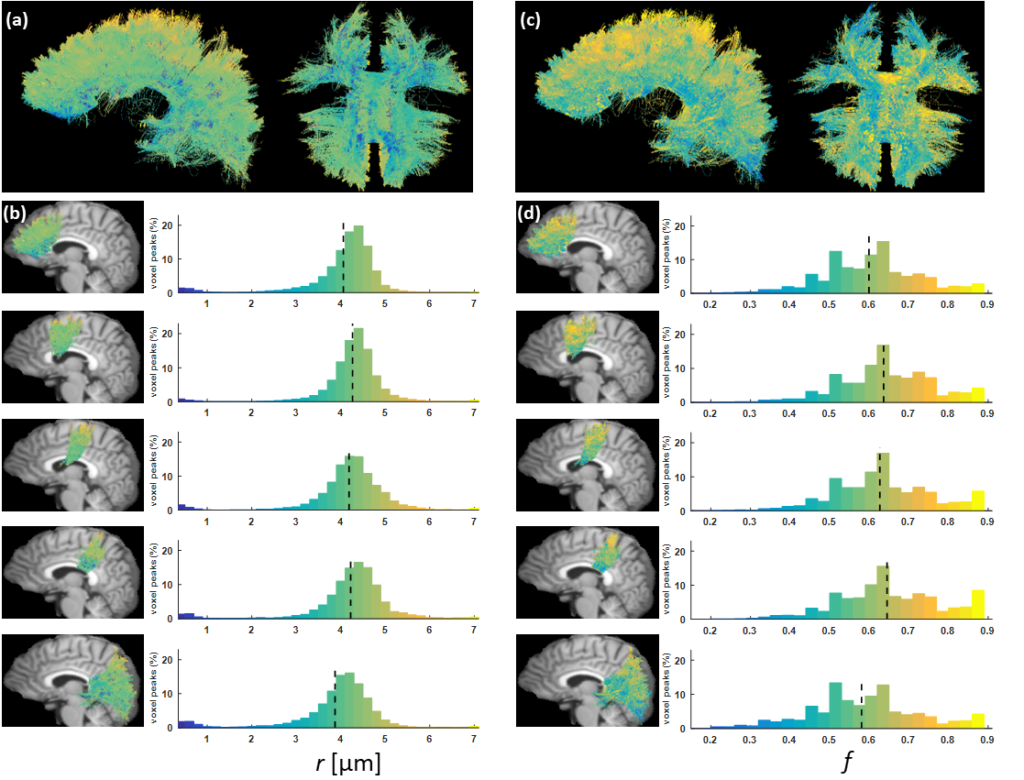